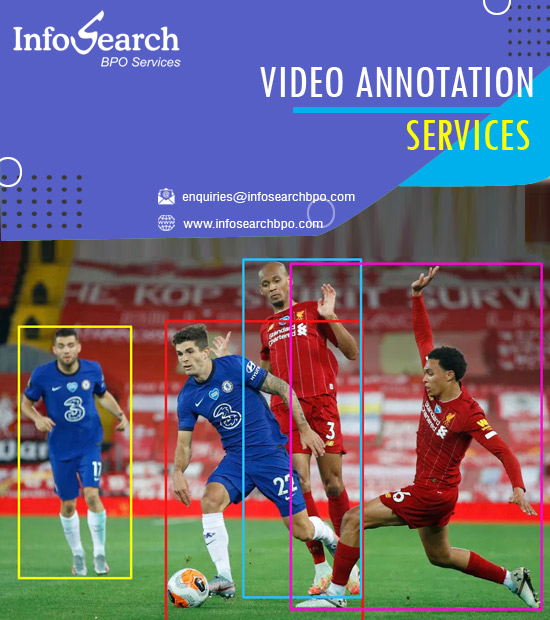
The video annotation process is a vital part of the technological application of different industries, in particular, machine learning, computer vision, entertainment, marketing, healthcare, etc. It encompasses those pertaining to the use of media assets associated with videos, for instance; having metadata, labels, or markers to provide context, understanding and schematization.
Infosearch BPO is a leading provider of various types of annotation services, including video annotation. Infosearch is an ISO-certified company and we follow data protection and GDPR compliance policies. We provide annotation services with over 400 full time employees who are trained in various types of annotations. We combine human annotations with advanced AI tools to deliver accurate results.
Here’s a simple yet comprehensive guide to video annotation:
Understanding Video Annotation:
In the context of video, annotation is all about labelling the different elements of the video so that the machine or a human can better comprehend it and therefore use it in their analysis, training, or interpretation.
Types of Video Annotation:
1. Object Annotation: Marking in particular those things in the frame which are of great importance and providing them with short descriptions in Latin. This is applicable for tasks that include object detection, tracking, and recognition, along with other multiple functions.
2. Action Annotation: Add text to the objects or people to let the user easily understand the action or activity. We highlight the importance of such analysis, as it becomes an inevitable step for action recognition and behavior analysis.
3. Temporal Annotation: Signaling special time marks within the video to note periods of time, the events, the transitions, and the changes. Such practice gives marketers useful information and helps them segment their video content to offer a personal touch.
4. Attribute Annotation: ‘Detailing objects or persons with unique attributes or traits in the video is one of the key factors of our interactive strategy. This may particularly consist of features such as colour, size, shape, etc.
5. Emotion Annotation: Discovering and outlining the emotional state of characters in the acted video. This, however, is very useful for tasks or purposes like delivering opinions and in developing affective computing.
6. Spatial Annotation: Assigning certain areas to definite points within the video frame. This is essential for a process in which sub-tasks like semantic segmentation and scene understanding can be accomplished.
Tools and Techniques:
1. Manual Annotation: Human annotators customarily employ the tools and software for the purpose of labeling done automatically on the objects, actions, or events in the frame of the video by taking manual approach.
2. Semi-Automated Annotation: Mixing up the manual annotation by technical methods will make the process quicker and easier. For example, this can involve identifying an initial annotation, which is after that humanizes it by the human annotators.
3. Automated Annotation: Applying computer vision algorithms and techniques through machine learning in order to annotate video data automatically based on predefined classifier features or models. However, this can increase the time and necessitate complex data preparation or verification.
Best Practices:
1. Quality Control: Ensure accurate, identical in style, and content-rich annotations through quality control measures like inter-annotator agreement checks and verification steps.
2. Scalability: Apply annotation tools and methods that can be used on a massive amount of data effectively.
3. Domain Expertise: Engage experts and stakeholders to guarantee that annotations reflect the key information, cover required objectives and meet specific targets.
4. Version Control: Maintain the iterable annotation of multiple sources for traceability of edits, cancellations, and updates down through the process of time in shared projects.
Applications:
1. Machine Learning: Examples of tasks for a vision system in a driverless car are: object detection, action recognition, and video classification by means of training and testing machine learning models.
2. Computer Vision: Intricate video content examination and the interpretations of which are used in applications such as surveillance, autonomous vehicles and augmented reality among others.
3. Entertainment: Development of gaming, virtual reality, as well as augmented reality applications, which are interactive and engaging.
4. Marketing: Through ways of customization and optimization of the video content for targeted advertisements, content recommendations, and audience engagement.
5. Healthcare: Data analysis of medical images, monitoring of patient behavior including prescription analytics, and assisting in diagnosis and treatment planning.
Challenges:
1. Annotation Cost: The manual annotation task may require too much time and money, most especially when the size of a video data set keeps on increasing.
2. Subjectivity: Annotating highly complex or ambiguous videos may be harder because the annotator can have varying points of views about the annotations provided therefore considerable variation can arise.
3. Data Privacy: Developing a framework for monitoring legal compliance requirements and ensuring data protection from any undesirable information entailed within video data.
4. Labeling Consistency: Creating and keeping the annotation consistency among the elements of annotation, for example, different annotators and annotation sessions, difficult specifically in collaborative projects.
Through the implementation of the aforementioned approach, data annotation of video data can be a useful tool to explore meaningful insights, bring about the creation of dependable machine learning models, and hence use the video-based applications across various domains. Infosearch provides all types of video annotation services that are discussed here. We combine human annotation with automated tools to give you accurate datasets for machine learning. Contact Infosearch for your video annotation requirements.
Recent Comments